Are you looking for the best OpenAI alternatives? If you’re wondering what the best options are, how they compare to OpenAI, and what criteria to consider when choosing the right one – this article is for you.
When researching OpenAI alternatives, you may be asking yourself questions such as: What is the best alternative to OpenAI? What are the differences between OpenAI and its alternatives? How do I choose the best OpenAI alternative for my needs?
The growth of AI and machine learning technologies has made it increasingly difficult for organizations to keep up with the latest advancements. Despite being a strong tool, OpenAI might not be the ideal choice for everyone. You can choose which one is best for you by exploring the many OpenAI choices.
This article provides insight into leading OpenAI alternatives, detailing their features and capabilities, and guiding criteria for selection, essential for developers in business software seeking advanced AI tools to enhance project outcomes and innovation.
What is OpenAI?
OpenAI is an AI (artificial intelligence) research lab founded in December 2015 by Elon Musk, Sam Altman, Ilya Sutskever, Greg Brockman, Wojciech Zaremba, and John Schulman. By researching and developing innovative technologies that can be used to benefit humanity, OpenAI aims to improve artificial intelligence. Intending to make AI accessible to everyone, OpenAI provides open-source tools and resources to help programmers and researchers develop AI-powered products.
What makes OpenAI different from other organizations? Compared to other AI organizations, OpenAI is different in several ways. First of all, OpenAI is open source, meaning everyone has access to their research results and tools. To develop their AI-driven products and bring them to market, companies and individuals can use OpenAI’s technology. OpenAI also has an outstanding team of researchers, engineers, and scientists who continue to push the boundaries of artificial intelligence research. Furthermore, OpenAI is dedicated to creating AI responsibly and ethically, guaranteeing that AI is safe and secure. The purpose of OpenAI in democratizing AI is to deliver the power of AI to everyone.
Exploring OpenAI alternatives is essential for business software developers seeking innovative AI solutions, offering a broader spectrum of tools and approaches to drive advancements in AI applications and enhance competitive edge in technology-driven markets.
DeepMind
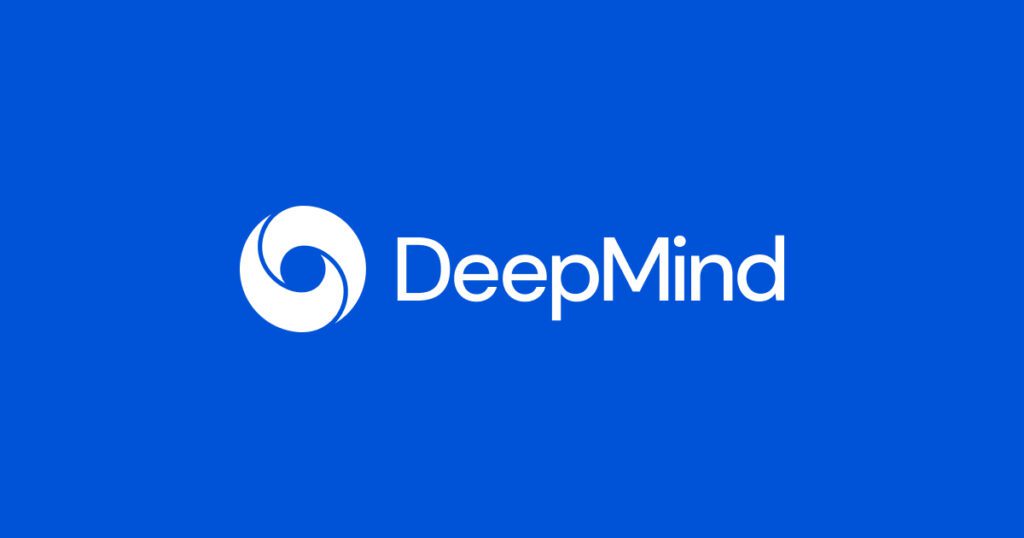
DeepMind is a UK-based AI research center that has achieved significant advances in the field of AI since its foundation in 2010. The goal of DeepMind is to apply AI to address the most critical issues facing the planet. Also, to apply artificial intelligence to tackle some of the world’s most difficult challenges, such as climate change and healthcare. Machine learning and other related approaches, such as reinforcement learning, and unsupervised learning, are the main topics of DeepMind’s research.
Usage
DeepMind Technologies is used to develop artificial intelligence (AI) systems for various tasks, such as natural language processing, image recognition, game playing, and robotics. It is also used to develop machine learning algorithms for data analysis, pattern recognition, and decision-making. DeepMind Technologies is used in various industries, such as healthcare, finance, and defense. DeepMind Technologies is utilized in healthcare for medical image analysis, clinical decision support, and healthcare system optimization. In finance, it is used for fraud detection, financial forecasting, and investment analysis. In defense, it is used to improve military operations, surveillance, and targeting.
Pros & Cons of DeepMind
Pros of DeepMind:
- DeepMind places great emphasis on research, making it a pioneer in the development of general-purpose AI systems.
- DeepMind’s AI solutions are closely interwoven with Google’s goods and services.
- There is close collaboration between DeepMind and partners in business and academia.
- DeepMind’s advancements in reinforcement learning techniques have improved the area of AI.
- A universal AI platform that is available to everyone.
Cons of DeepMind:
- DeepMind’s algorithms are not open source, thus they can only be used with Google goods and services.
- DeepMind’s AI solutions are still in the early phases of development, thus they may be less effective or efficient than more established AI solutions.
- DeepMind’s AI solutions may be too sophisticated for certain people to comprehend and use.
IBM Watson
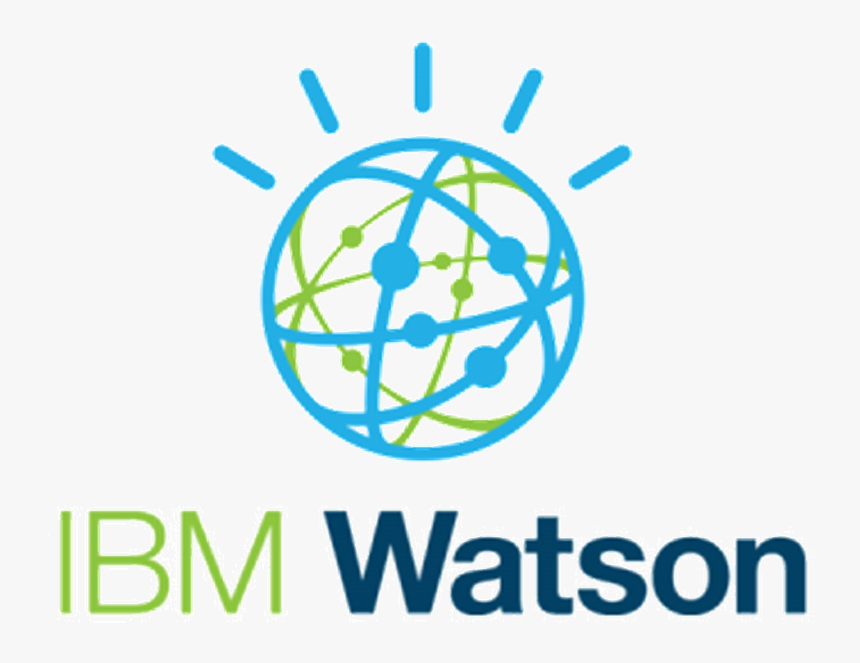
IBM Watson is an AI platform developed by IBM that enables the development of cognitive applications. It uses natural language processing and machine learning to understand complex unstructured data and is based on data-driven algorithms. Its main purpose is to provide users with an intuitive and automated way to gain insights from their data. IBM Watson can be used in a variety of fields, including healthcare, finance, and retail. Watson provides natural language processing, image recognition, speech recognition, and other cognitive services that can help businesses make better decisions and improve the customer experience.
Usage
Healthcare, banking, retail, and education are just a few of the industries where IBM Watson could be used. In medicine, Watson can be used to diagnose diseases, determine the best course of action and even detect cancer tumors in their early stages. In finance, it can examine financial data, identify trends and detect fraud. In retail, it may be applied to consumer behavior analysis, product recommendations, and individualized customer experiences. In the field of education, it may be used to track down students who are in danger of failing classes, design individualized lessons, and provide instructors with individualized feedback.
Pros & Cons of IBM Watson
Pros of IBM Watson
- Highly accurate and efficient, allowing organizations to gain insights from their data quickly and accurately.
- Can be used to analyze complex unstructured data, such as images, videos, and natural language.
- Can be used in a variety of fields, such as healthcare, finance, retail, and education.
- Can help organizations make better decisions, improve customer experiences, and increase efficiency.
Cons of IBM Watson
- Expensive and requires a lot of computing power.
- Can be difficult to set up and maintain.
- Can be difficult to integrate into existing systems.
- May not always be able to provide accurate results, as it relies on machine learning algorithms.
Microsoft Azure
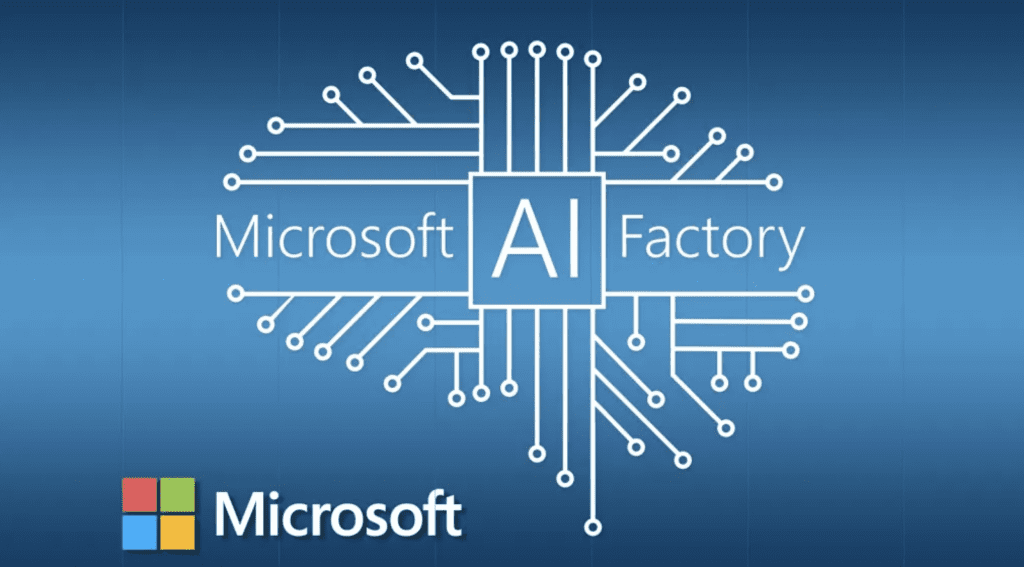
The Microsoft Azure cloud computing platform was created by Microsoft, and it includes a variety of cloud services, like processing, storage, networking, analytics, and the creation of mobile and web apps. The Microsoft Azure cloud computing platform is designed to help businesses quickly install and manage cloud apps and services. Microsoft Azure provides a user-friendly platform for programmers and businesses to develop, manage, and deploy cloud-based apps and services. It also provides a variety of services such as big data analytics, the Internet of Things (IoT), artificial intelligence (AI), and machine learning, allowing businesses to optimize their operations and get insights from their data.
Microsoft Azure can be used for a variety of AI-related tasks:
- Image recognition and classification
- Natural language processing
- Speech recognition
- Text analytics
- Machine learning
- Predictive analytics
- Robotics
- Autonomous systems
Pros & Cons of Microsoft Azure
Pros of Microsoft Azure
- Highly scalable and can be used for a variety of tasks.
- Provides a wide range of services and tools for developers.
- Easy to use and can be integrated with existing systems.
- Cost-effective and provides a secure environment.
Cons of Microsoft Azure
- Complex and can be difficult to set up and manage.
- Requires a lot of expertise to use effectively.
- Not always provide the best performance for some tasks.
Google Cloud AI
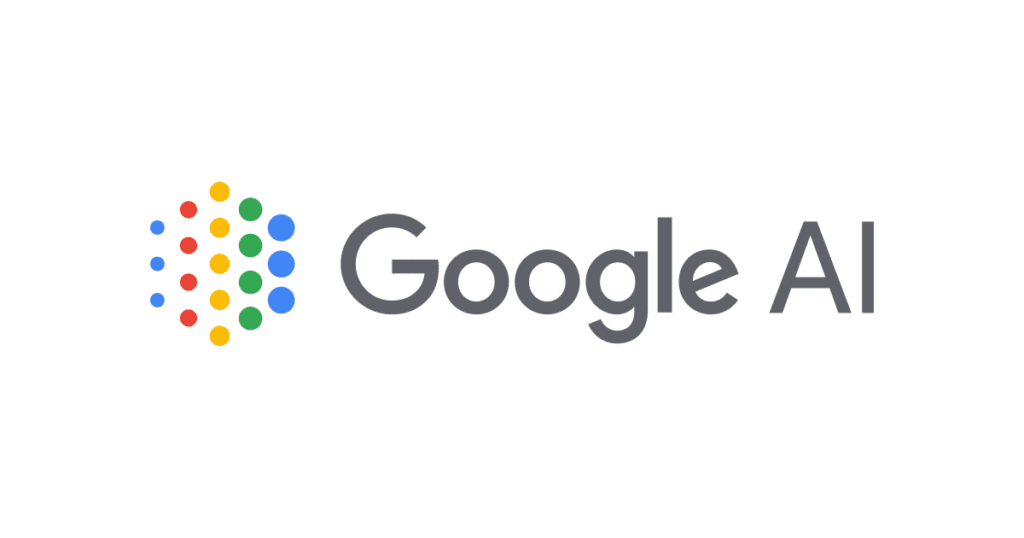
Google Cloud AI is a collection of AI services and tools for developing AI applications. It provides pre-trained models and services for developing intelligent apps that can respond to user input, forecast outcomes, and recognize speech. Google Cloud AI is designed to make it simpler for programmers to construct AI apps that can solve challenging issues and enhance user experiences.
Usage
Building apps that comprehend natural language, recognize pictures, process audio and video, and identify things in photographs is the goal of Google Cloud AI. Applications that promote goods, advise activities and automate customer service chores can all be created using it. Insights and analytics may be produced as a way to streamline business processes and make informed decisions.
Pros & Cons of Google Cloud AI
Pros of Google Cloud AI
- Provides a wide range of services and tools that can be used to create powerful AI applications.
- The services are highly scalable and can be used to create applications that can handle large volumes of data.
- Cost-effective and can be used to create applications that can handle large volumes of data without incurring high costs.
Cons of Google Cloud AI
- Google Cloud AI can be complex and difficult to use for developers who are new to the platform.
- Limited support for developers who are new to the platform.
Amazon Machine Learning
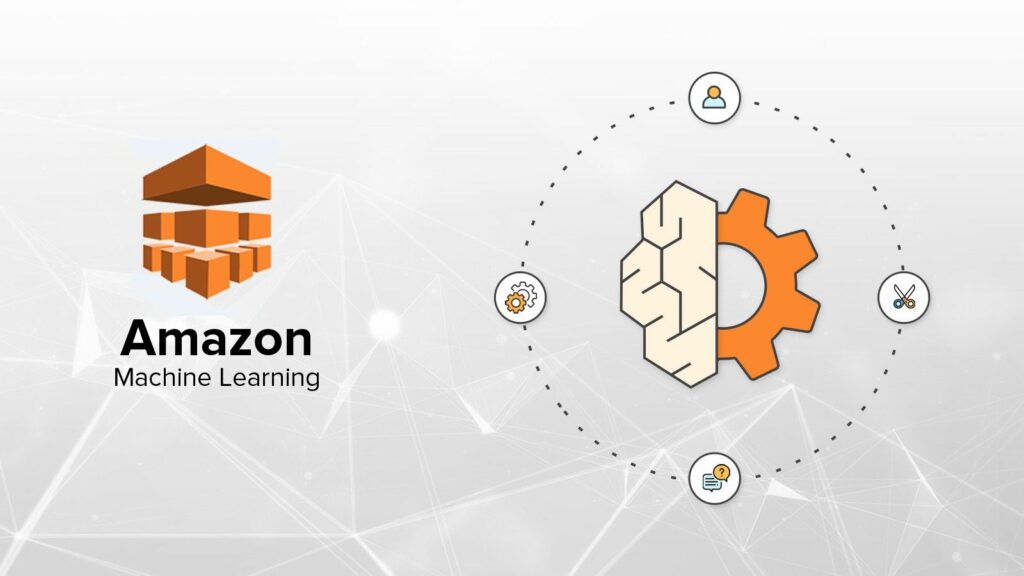
Amazon Machine Learning is a cloud-based AI service and product package that allows developers to create predictive applications. It gives you the tools and techniques you need to create apps that can analyze data, spot patterns, and make predictions. Amazon Machine Learning is made to help developers create software that anticipates consumer behavior, recommends products or services, looks for fraud or other abnormalities, and recognizes trends. Software that automates customer service chores, uncovers abnormalities in medical data, or draws insights from huge datasets may all be made using this technique.
Pros and cons of Amazon Machine Learning
Pros of Amazon Machine Learning
- Provides an intuitive interface that makes it easy for developers to create predictive applications.
- Scalability: can be used to create applications that can handle large volumes of data without incurring high costs.
- Cost-effective and can be used to create powerful applications without incurring high costs.
Cons of Amazon Machine Learning
- Limited support for developers who are new to the platform.
- Limited flexibility and customization options for developers.
NVIDIA DGX
NVIDIA DGX is a high-performance computing system designed to meet the needs of data-intensive workloads like deep learning. It is made to speed up AI and machine learning workflows, enabling users to create deep learning models quickly. Along with completely integrated and deep learning-optimized software, it consists of a group of potent GPU servers powered by NVIDIA. The most effective deep learning and AI development platforms are provided by NVIDIA DGX, which enables customers to rapidly and effectively develop, deploy, and manage their applications.
Usage
NVIDIA DGX is primarily designed to speed up AI and machine learning processes. Rapid deep learning model development, application deployment and management, and high-performance computer operations are all possible with it. Data scientists may experiment with their deep-learning models more quickly and effectively by using NVIDIA DGX to build virtualized computing environments.
Pros & Cons of NVIDIA DGX
Pros of NVIDIA DGX
- High performance in deep learning and AI development.
- Fully integrated and optimized software for deep learning.
- Easy to use and deploy.
- Virtualized computing environment for data scientists.
Cons of NVIDIA DGX
- Expensive.
- May not be suitable for all types of data-intensive workloads.
Intel AI
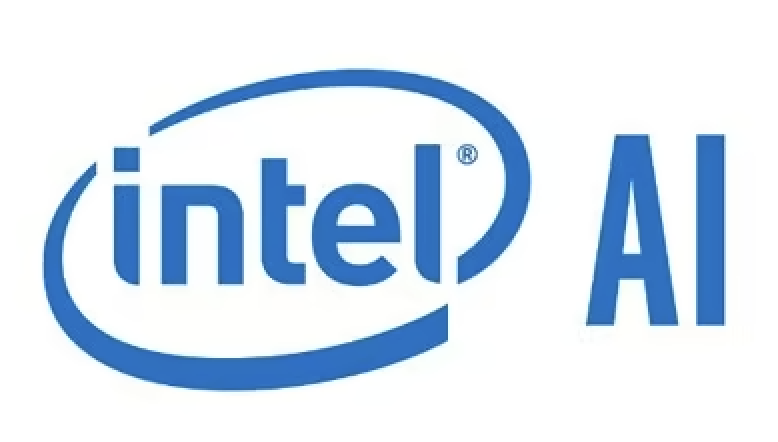
Intel AI is a suite of hardware and software solutions for artificial intelligence (AI) designed to deliver performance and flexibility for AI workloads. Intel AI solutions provide the computing power to run deep learning and other AI models, as well as advanced analytics and real-time insights. Intel AI solutions are designed to be used in a wide variety of applications, such as autonomous driving, healthcare, and robotics. Intel AI solutions are optimized for Intel-based platforms and are fully integrated with Intel architecture. The main purpose of Intel AI is to provide the computing power and flexibility needed to run AI models, analytics, and real-time insights.
Usage
Intel AI can be used in a variety of applications, including autonomous driving, healthcare, robotics, and more. Intel AI solutions can be used to train deep learning models, run analytics, and generate real-time insights. Intel AI solutions can also be used to develop and deploy AI applications on Intel-based platforms.
Pros & Cons of Intel AI
Pros of Intel AI
- Optimized for Intel-based platforms.
- Fully integrated with Intel architecture.
- High performance in deep learning and AI.
- Flexibility to run a variety of AI applications.
Cons of Intel AI
- Not as widely available as other AI solutions.
- Potentially more expensive than other AI solutions.
Apple Core ML
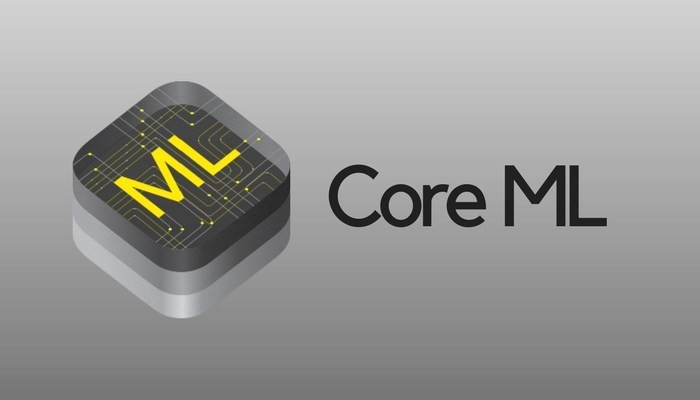
Apple Core ML is a machine learning platform developed by Apple to enable developers to quickly and easily integrate machine learning models into their iOS, macOS, watchOS, and tvOS apps. Core ML allows developers to take advantage of the power of machine learning without having to write complex algorithms or deep learning models. Core ML leverages the power of Apple’s hardware and software to enable developers to quickly create and deploy machine learning models for their apps. The main purpose of Apple Core ML is to make it easy for developers to integrate machine learning into their apps.
Usage
Apple Core ML can be used to quickly create and deploy machine learning models for iOS, macOS, watchOS, and tvOS apps. Core ML models can be used for a variety of tasks, including image recognition, natural language processing, and more. Core ML also allows developers to take advantage of the power of Apple’s hardware and software to optimize their models for performance and accuracy.
Pros & Cons of Apple Core ML
Pros of Apple Core ML
- Quick and easy to integrate machine learning into apps.
- Leverages the power of Apple’s hardware and software.
- Optimizes models for performance and accuracy.
- Variety of tasks supported, including image recognition and natural language processing.
Cons of Apple Core ML
- Limited to Apple-specific platforms.
- May not be suitable for all types of machine-learning tasks.
- Dependent on Apple for updates and bug fixes.
H2O.ai
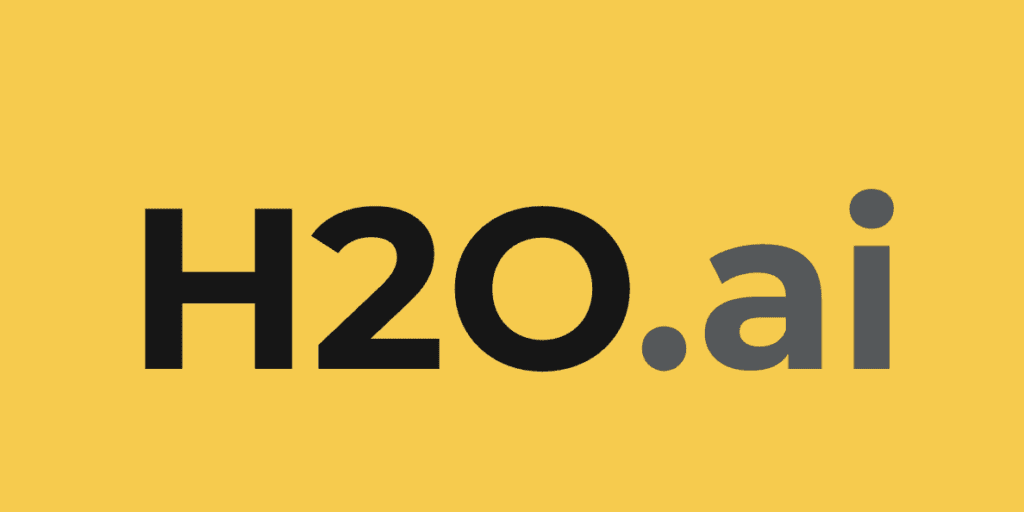
H2O.ai is an open-source platform for machine learning and artificial intelligence. It provides a range of tools and technologies for data scientists to quickly and easily develop, deploy, and manage machine learning models. H2O.ai is designed to work with a wide variety of data sources, including relational databases, text files, spreadsheets, and more. The main purpose of H2O.ai is to enable data scientists to quickly and easily develop, deploy, and manage machine learning models.
Usage
H2O.ai can be used to develop and deploy machine learning models for a wide variety of applications. H2O.ai provides a range of tools and technologies for data scientists to quickly and easily develop, deploy, and manage their models. H2O.ai can also be used to create virtualized computing environments for data scientists, allowing them to experiment more quickly and efficiently with their models.
Pros & Cons of H2O.ai
Pros of H2O.ai
- Open-source platform
- Easy to use and deploy
- Works with a wide variety of data sources
- Ability to create virtualized computing environments
Cons of H2O.ai
- May not be suitable for all types of machine-learning tasks
- Limited support for certain data sources
- Limited scalability for larger datasets
OpenCV
OpenCV is an open-source computer vision library for real-time image and video processing. It provides a wide range of algorithms and functions for image and video analysis, including feature detection, object detection, and tracking. OpenCV is designed to be user-friendly and efficient, allowing developers to quickly and easily create complex applications for vision-based systems. The main purpose of OpenCV is to provide developers with a powerful and easy-to-use library for real-time image and video processing.
Usage
OpenCV can be used to create a wide range of applications for vision-based systems. It can be used for feature detection, object detection, and tracking, as well as a variety of other image and video processing tasks. OpenCV can also be used to create virtualized computing environments for developers, allowing them to experiment quickly and efficiently with their applications.
Pros and cons of OpenCV
Pros of OpenCV
- User-friendly and efficient.
- Open-source library.
- Wide range of algorithms and functions.
- Ability to create virtualized computing environments.
Cons of OpenCV
- Limited support for a certain image and video formats.
- May not be suitable for all types of vision-based systems.
- Can be difficult to debug and optimize code.
Summing Up
These are some of the top 10+ OpenAI alternatives that you should consider for your AI projects. Each of these AI research facilities has advantages and disadvantages, so you should examine your alternatives before picking which one to utilize. We hope that this article has helped you understand the many alternatives available as well as the benefits and drawbacks of each.
Comments